Using machine learning to spatially classify current technologies used for cooking in developing countries
Worldwide, about 2.4 billion people still lack access to clean cooking fuels. This forces people to rely on traditional fuels, which has been shown to have severe consequences on their health, cause environmental pollution, degrade ecosystems and impact livelihoods of mainly women and children. One of the issues around this problem is the lack of understanding of the behavioural factors driving the choice of cooking technology in a household. In order to bridge this knowledge-gap, this master thesis will develop machine learning models to spatially classify an area with the most probable technologies currently used for cooking.
Background
Energy for cooking is considered essential in achieving modern energy access. Despite this, almost 2.4 billion people still lack access to clean cooking, most of them living in rural areas of industrializing countries in sub-Saharan Africa, developing Asia and parts of central- and south-America (IEA, 2020b). In order to mitigate the shortcomings of clean cooking solutions, traditional energy sources tend to be used, which can harm people's health (Fuso Nerini et al., 2017). IEA estimates that in developing Asia (excluding India and China), 0.6 million people died due to household air pollution caused by using non-modern cooking solutions (IEA, 2020a). Furthermore, the collection of traditional fuels tends to be either costly or time-consuming and, in many instances, the burden of fuel collection falls on children and women (WHO, 2016). Reaching universal access to clean cooking solutions is also part of SDG 7 (indicator 7.1.2 Proportion of population with primary reliance on clean fuels and technology) (UN, 2020). SDG 7 has previously been connected to many other SDGs (Fuso Nerini et al., 2018). By reducing the under-the-age-of-five mortality rate and the rate of early deaths due to air pollution, clean cooking can contribute to SDG3 (Health and wellbeing). By alleviating women and children from the burden of fuel collection, it can contribute towards SDG5 (gender equality). Furthermore, it can also contribute to SDG 13 (climate action) and SDG15 (reducing deforestation) (Rosenthal et al., 2018).
In order to enable policy-makers to create sensible strategies that achieve a successful penetration of clean cooking sources worldwide, there is the need to understand which socio-economic and biophysical factors can influence the choice of energy source for cooking (Khavari et al., 2023). Factors such as income, household size, age, gender, accessibility to free sources (e.g. wood or animal dung), electrification, and climate, among others, can significantly influence such choices.
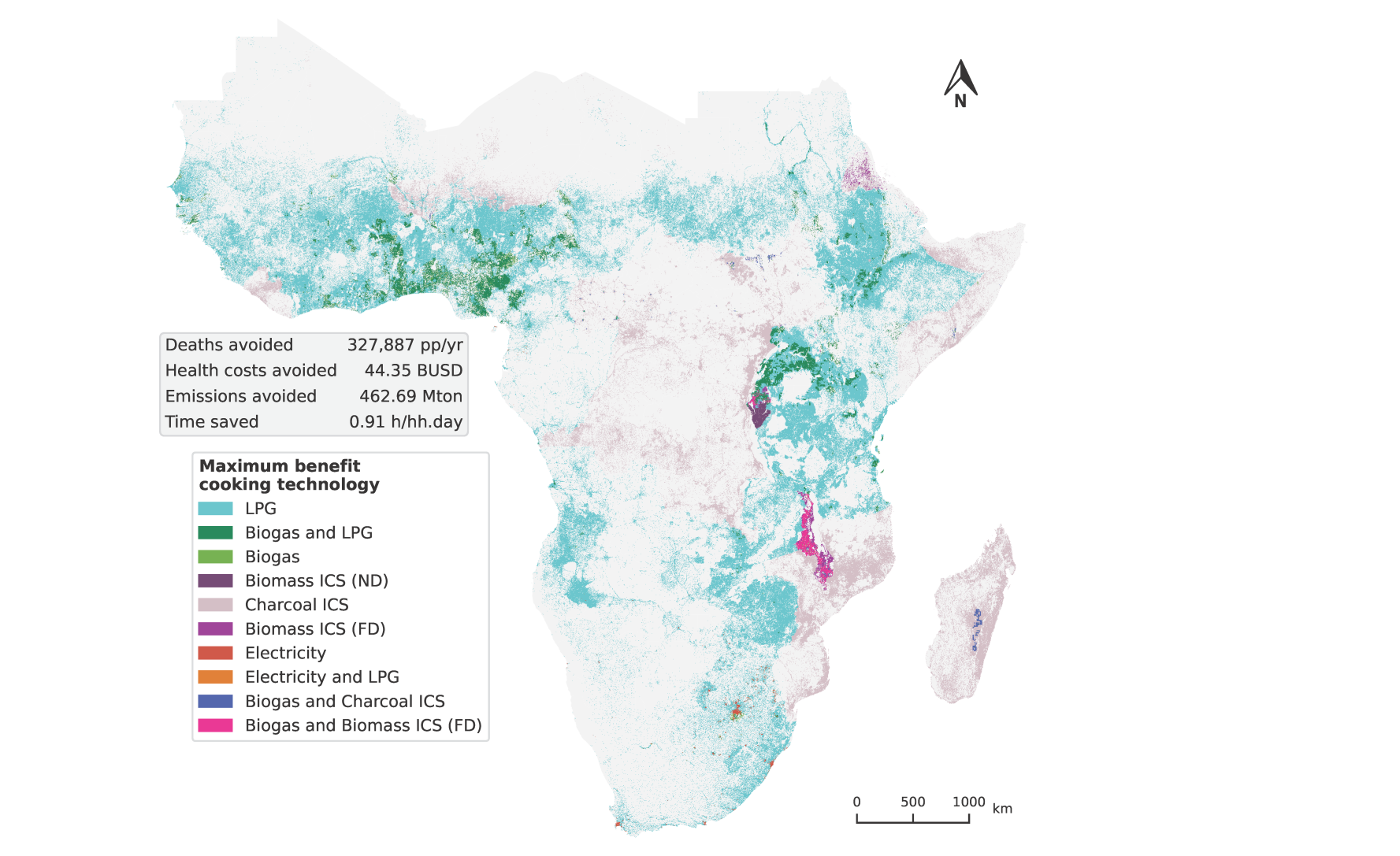
Task description
The student(s) (up to 2) task will be to conduct a two-step literature review.
-
To understand the most relevant factors affecting the choice of energy source for cooking. This review will start with a general perspective and evolve into a country case study.
-
To understand how machine learning models have been used for geospatial modelling of energy systems, social science applications, and specific applications of clean cooking modelling if any.
After finishing the literature review and drawing meaningful conclusions on the knowledge gaps, the students will use one of the Multi-Tier Framework (MTF) surveys to explore correlations between selected variables for the country case. The MTF is an initiative that redefines how energy access is measured; it goes beyond the traditional binary measure of "connected or not connected" for electricity access and "solid vs nonsolid fuels" for cooking. Quantitative measures such as the Pearson correlation coefficient or the chi-square statistic can be used to identify correlations between the variables based on the survey datasets. Then, the students will use data from the Multi-Tier Framework (MTF) related to the correlated variables to develop and train a supervised machine learning algorithm. Finally, this trained model will be used to classify each square kilometre of the country case in terms of the current most probable technology used for cooking using geospatial datasets.
If the work is of good quality and the student(s) are interested, the research project will be designed to be suitable for a peer-reviewed publication in a high-quality journal.
Learning outcomes
-
Understanding the importance of clean cooking for reaching SDG7 and its potential impact in sustainability (other SDGs). Comprehend the socio-economic and biophysical factors that can influence the choice of energy source for cooking and their importance for a successful clean cooking transition.
-
General knowledge of supervised machine learning algorithms and their use for classification of geospatial data.
-
Apply supervised machine learning algorithms on a real-life case study.
-
Basic knowledge of Geographic Information Systems (GIS) and its use for energy systems modelling.
Criteria for evaluation
Critical criteria in the complete work, method development and metric for the final assessment are:
-
Fulfilment of the ILOs for Master Thesis at KTH's ITM School;
-
The student's initiative and independence in developing the overall research design;
-
A critical discussion of the assumptions and results;
-
Consideration of the literature.
-
The ability to communicate the results of scientific work clearly and coherently.
Prerequisites
This project is a fit for students that are comfortable working with multidisciplinary subject. Basic previous knowledge on how to conduct a literature review is required. Previous knowledge on supervised machine learning algorithms is advised. Skills in Python programing are preferred.
Specialization track
Transformation of Energy System (TES)
Division/Department
Division of Energy System s – Department of Energy Technology
Research areas:
Duration
5-6 months, start January 2025.
How to apply
Send an email expressing your interest in the topic to Camilo Ramirez (camilorg@kth.se) and Manuel Enrique Salas (mess@kth.se).
Supervision
Examiner
References
- Fuso Nerini, F., Tomei, J., To, L. S., Bisaga, I., Parikh, P., Black, M., Borrion, A., Spataru, C., Castán Broto, V., Anandarajah, G., Milligan, B., & Mulugetta, Y. (2018). Mapping synergies and trade-offs between energy and the Sustainable Development Goals. Nature Energy, 3(1), 10–15. https://doi.org/10.1038/s41560-017-0036-5
- IEA. (2020a). Access to clean cooking and premature deaths from household air pollution in sub-Saharan Africa and Asia, 2010-2018. IEA. https://www.iea.org/data-and-statistics/charts/access-to-clean-cooking-and-premature-deaths-from-household-air-pollution-in-sub-saharan-africa-and-asia-2010-2018
- IEA. (2020b). SDG7: Data and Projections. IEA. https://www.iea.org/reports/sdg7-data-and-projections
- Khavari, B., Ramirez, C., Jeuland, M., & Fuso Nerini, F. (2023). A geospatial approach to understanding clean cooking challenges in sub-Saharan Africa. Nature Sustainability. https://www.researchsquare.com/article/rs-1650097/v1
- Nerini, F. F., Ray, C., & Boulkaid, Y. (2017). The cost of cooking a meal. The case of Nyeri County, Kenya. Environmental Research Letters, 12(6), 065007. https://doi.org/10.1088/1748-9326/aa6fd0
- Rosenthal, J., Quinn, A., Grieshop, A. P., Pillarisetti, A., & Glass, R. I. (2018). Clean cooking and the SDGs: Integrated analytical approaches to guide energy interventions for health and environment goals. Energy for Sustainable Development, 42, 152–159. https://doi.org/10.1016/j.esd.2017.11.003
- UN. (2020). SDG7 indicator 7.1.2: Proportion of population with primary reliance on clean fuels and technology. United Nations. https://sdgs.un.org/goals/goal7
- WHO. (2016). Burning Opportunity: Clean Household Energy for Health, Sustainable Development, and Wellbeing of Women and Children (p. 113). World Health Organization.